The Era of the Invisible Workforce and Its Future
Artificial intelligence today stands as the symbol of humanity’s most transformative wave of development. It is often associated with
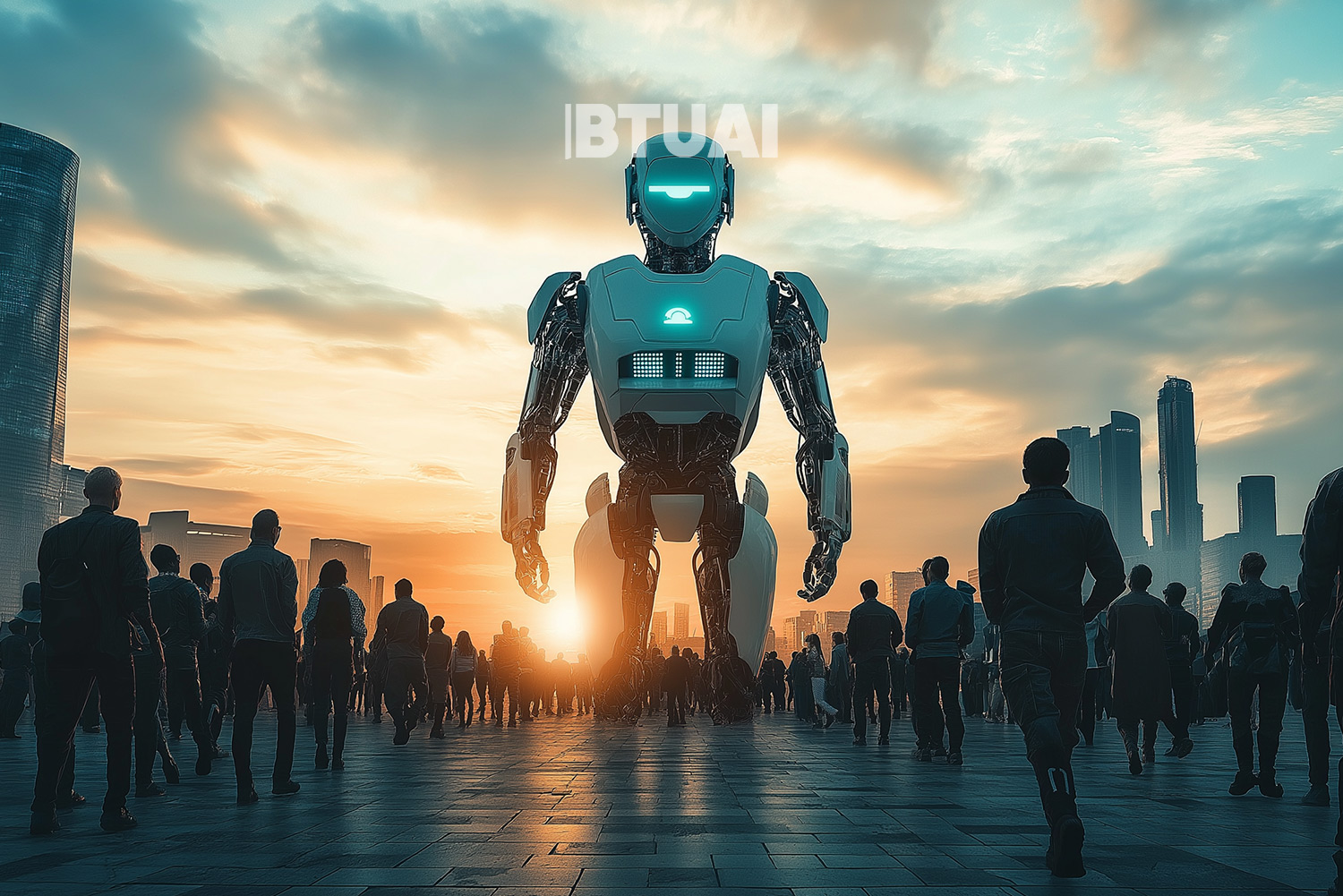
Artificial intelligence today stands as the symbol of humanity’s most transformative wave of development. It is often associated with high-end technologies, robots, and algorithms that seemingly create impressive results without human involvement. However, the reality of AI is far more complex — behind it stands a vast, largely invisible global workforce that plays a critical role in training, correcting, and expanding these systems.
This workforce consists of individuals who, for years, have labeled objects in images, annotated audio files, written texts and code, checked model outputs, and assessed their accuracy. For some, it was an opportunity to earn extra income; for others, particularly in developing countries, it became the sole source of livelihood.
For modern models to understand what “braking” means in motion, or to decipher human speech, they must have access to thousands of manually analyzed and properly annotated examples. These processes rely heavily on human labor — labor that is rarely mentioned in presentations or represented in investment documents.
For instance, American researcher Fei-Fei Li, often called the “midwife of AI,” in the 2000s gathered hundreds of thousands of annotators worldwide through Amazon’s Mechanical Turk platform to create ImageNet — the largest image database that made today’s visual AI development possible.
Yet it seems this model is now changing. New AI systems, particularly large language models (LLMs), are increasingly less reliant on manually annotated data. Companies like OpenAI and other leading labs are turning to self-supervised algorithms, reinforcement learning, and synthetic data.
Nevertheless, the human role is not disappearing — it is evolving. Annotators today are expected to possess software skills, specialized domain knowledge, and analytical abilities. It is no longer about simply distinguishing between cats and dogs in photos — they must evaluate algorithmic outputs, explain why a particular answer is correct or incorrect, and often articulate better responses themselves.
As the annotator workforce in India alone could exceed 1 million people by 2030, generating up to $7 billion in revenue, the global demand is growing for workers who know foreign languages and can prepare data that makes systems linguistically and culturally more multinational.
But risks persist. As tech giants increasingly invest in “non-magical,” self-learning systems, the value of human-driven invisible labor may diminish. Many workers face intense monitoring, low wages, and their contributions are often obscured — presented instead as the “automatic” achievements of technology.
Yet, as this article emphasizes, a model’s ultimate performance, accuracy, and ethicality still depend on human participation. It was through human feedback that ChatGPT’s response selection and safety filters were developed. And it turns out that the linguistic “character” of a model is often shaped by the regions from which its annotators come — African English expressions, Asian syntactic constructions, and other linguistic imprints subtly shape AI’s language.
This process more closely resembles raising a child than deploying a piece of technology. Just as a child needs not only parents but an entire community, so too does artificial intelligence require human diversity, knowledge, and ethical stewardship. A future where machines learn without people is still distant. Until that moment arrives, humans will remain the most critical part of these systems — sometimes invisible, but always essential.
Prepared based on materials from nytimes.com